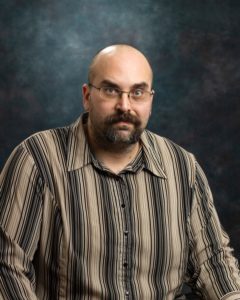
Omar Demerdash
Research Staff
Email: [email protected]
Work: 865.241.7687
Address:
1 Bethel Valley Rd.
Bldg. 8630, B-230
Oak Ridge, TN 37831-6309
WebsitesLinkedIn: https://www.linkedin.com/in/omar-demerdash-258160133 | |||
Education and BiographyPostdoctoral Scholar in Chemistry, University of California-Berkeley, 2013-2017 |
Research Interests
Developing a quantitative understanding of the structure, function, and dynamics of biological macromolecules, the interactions among them, and how such interactions modulate protein function is at the heart of a molecular-level understanding of all of biology. From a computational standpoint, these problems have traditionally been investigated using algorithms based on first-principles physical models. However, owing to underlying approximations and computational cost of such models, there exists some difficulty in making accurate predictions that engender synergy with experimental investigations. An approach towards realizing computational models that yield accurate predictions is to combine physics-based models with machine learning (ML). Therefore, the focus of my work is development of such hybrid physics/ML models and applications to problems of interest to BER.
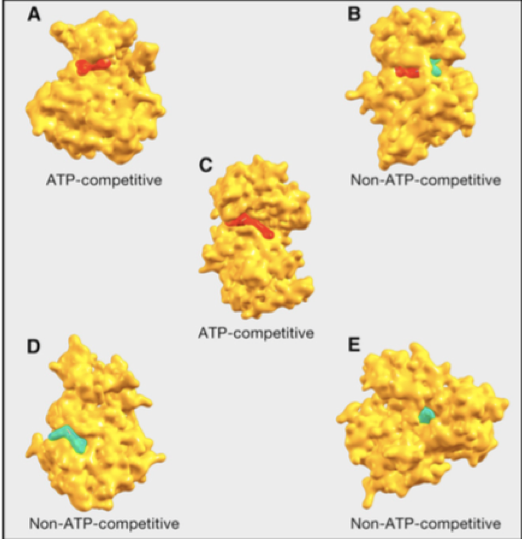
This work has proceeded along several fronts. I have developed ML models for the prediction of protein-ligand binding and applied those models to systems underlying plant-microbe symbiosis and SARS-Cov-2 virulence. To address the problem of how binding modulates the protein function, I am also working on using convolutional neural networks to predict the allosteric state of a protein. Along the same theme of predicting molecular interactions and their functional consequences, colleagues and I in the Biofuels SFA are using ML to predict interactions of lignocellulosic biomass with organic solvents with the ultimate goal of predicting which solvents deconstruct biomass most efficiently so that lignin, cellulose, and hemicellulose can be valorized as part of a green economy with a low carbon footprint.
I have also developed a technique where experimental small-angle scattering is used to tune the approximate potential energy models that are used in molecular dynamics. This has been applied to intrinsically disordered proteins and multicomponent lipid membranes.
Lastly and most recently, I have applied a combination of ML and physics to problems in enzyme engineering, including predicting product yields of diverse panels of putative enzymes and predicting the effects of site mutagenesis on enzyme function. The ultimate goal of this work is to break down and upcycle plastics using engineered enzymes, as plastics have become a significant pollutant owing to their inability to be recycled by the current state of the art.
Publications
- Mohan M, Demerdash O, Simmons BA, Smith JC, Kidder MK, Singh S. Accurate prediction of carbon dioxide capture by deep eutectic solvents using quantum chemistry and a neural network. Green Chemistry, 2023, 25: 3475-3492 (DOI: 10.1039/d2gc04425k).
- Cope KR, Prates ET, Miller JI, Demerdash ONA, Shah M, Kainer D, Cliff A, Sullivan KA, Cashman M, Lane M, Matthiadis A, Labbe J, Tschaplinski TJ, Jacobson DA, Kalluri UC. Exploring the role of plant lysin motif receptor-like kinases in regulating plant-microbe interactions in the bioenergy crop Populus. Computational and Structural Biotechnology Journal, 2023, 21: 1122-1139 (DOI: 10.1016/j.csbj.2022.12.052).
- Hameedi MA, Prates ET, Garvin MR, Mathews II, Amos BK, Demerdash O, Bechthold M, Iyer M, Rahighi S, Kneller DW, Kovalevsky A, Irle S, Vuong V-Q, Mitchell JC, Labbe A, Galanie S, Wakatsuki S, Jacobson D. Structural and functional characterization of NEMO cleavage by SARS-CoV-2 3CLpro. Nature Communications, 2022, 13: 5285 (DOI: 10.1038/s41467-022-32922-9).
- Demerdash O. Using diverse potentials for the development of improved machine learning models for protein-ligand affinity and docking pose prediction. Journal of Computer-Aided Molecular Design, 2021, 35: 1095-1123 (DOI: 10.1007/s10822-021-00423-4).
- Demerdash O, Shrestha UR, Petridis L, Smith JC, Mitchell JC, Ramanathan A. Using small-angle scattering data and parametric machine learning to optimize force field parameters for intrinsically disordered proteins. Frontiers in Molecular Biosciences, 2019, 6: 64 (DOI: 10.3389/fmolb.2019.00064).